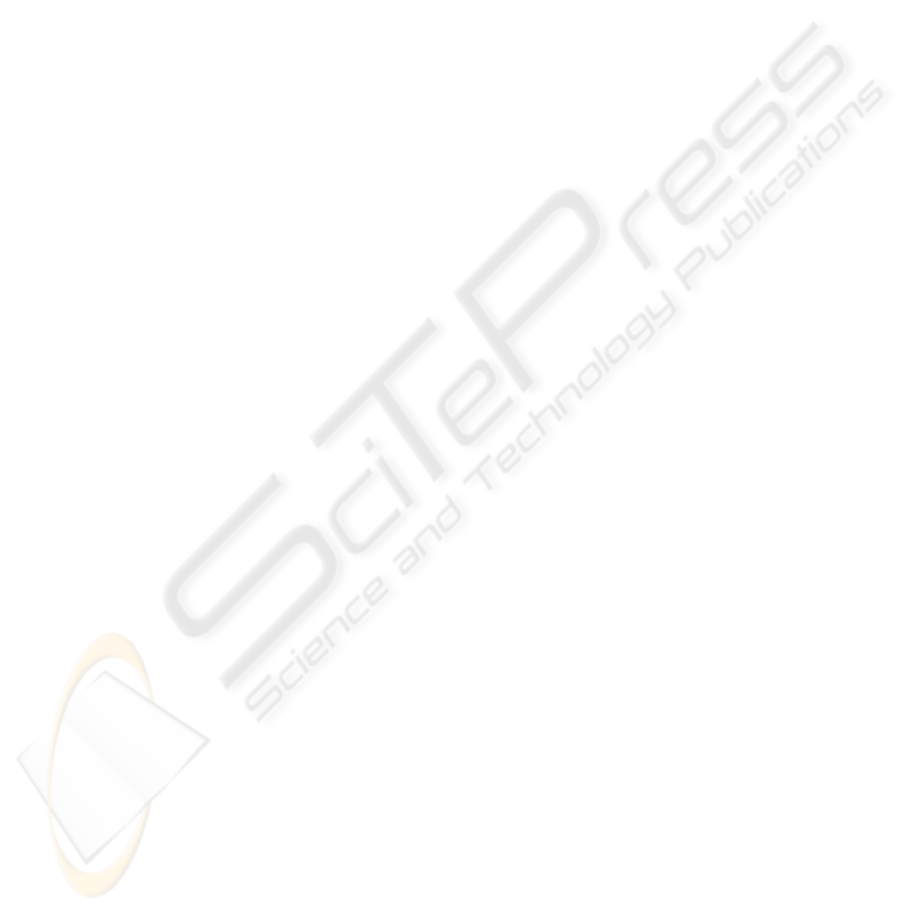
To verify the thesis we used GradeStat for
constructing tables of ARs. AR is the name given in
(Kowalczyk et al., 2004) to the concentration index;
it has a representation as an area contained in the
unit square. AR’s value for a material determines the
extent to which the material is dissimilar to the
pattern material in the set of features. The greater the
|AR|, the greater the dissimilarity between those two
materials. For simplicity, from now on we use AR
instead of |AR|.
We performed this analysis on a subset of the
population – we considered 37 out of the 56
materials that were evaluated by the respondents
(because only for them the respondents estimated the
time ratio for the level I elements). The set of
features included 4 features for the time ratios of the
level I elements (i.e., Introduction, Main content,
Summary, and Evaluation). Figure 3 shows the chart
of ARs, where OX is for materials ordered by their
average marks, and OY is for the ARs.
In the figure we can see that the results are quite
different even in the same groups (i.e., for the same
marks), but there is a clear trend of descending
values of ARs for subsequent groups. We can
conclude that even though it is rather difficult to
estimate the time ratios for the level I elements in
the case of e-learning materials (consequently, such
ratios are not a perfect quality measure for e-
learning materials), the descending trend of the ARs
and the average ARs makes the ratios a good partial
measure of the quality of e-learning materials. So we
decided to replace the four time ratio features with
one time_AR feature that says how close the time
ratios for the level I elements of a given material are
to the corresponding time ratios of the pattern
material.
3.3 Influence of the Correct Didactic
Structure of an e-Learning
Material on its Quality
In this section we will deal with the thesis that
following the recommendations of traditional
(paper) learning materials experts (in particular,
keeping the structure of such materials) is beneficial
also in the case of e-learning materials, that is, it
improves their quality. Furthermore, the existence of
specific elements (identified by experts), the
assessment of the quality of each such element, and
the time ratio for the level I elements can be used as
partial measures for the quality.
To verify the thesis we analyzed three
populations of materials. The first population was
comprised of all the 56 materials evaluated by the
respondents. The multiplicity of the set of features
was 40: for each of the 20 elements analyzed in the
questionnaire we considered both its existence and
its mark (for the level II elements we considered
either the marks by the respondents or 0 if there was
no such mark; for the level I elements we considered
the average marks based on the respondents’
subjective marks for the level II elements). In this
part of our analysis we did not take into account the
time_AR feature, because the respondents estimated
the time ratios only for 37 materials.
Figure 4 shows the ARs for this population,
where OX is for the identification numbers of the
materials that are ordered and grouped by their
average marks; OY is for the values of the ARs.
In the chart we can see a descending trend: the
smaller the average AR, the better the marks of a
given material. In each of the groups (for subsequent
average marks) we can clearly see that the results are
quite different – there are materials for which the
value of AR strongly deviates from the average
value in their group. Thus, in the next phase of our
analysis we took into consideration only those
materials for which the difference between their
average mark and their subjective mark is at most 2
standard deviations; there were 30 such materials.
We constructed this new population of materials and
computed ARs for it; the chart is in Figure 5.
As before, we can see a descending trend: the
smaller the average AR, the better the marks of a
given material, but this time the differences between
the results in each group are much smaller, probably
because the credibility of the data is greater. Hence,
we decided to increase the credibility even more by
constructing a population of only such materials for
which: (1) as before, the difference between their
average marks and their subjective marks was at
most 2 standard deviations; (2) the respondents
estimated the time ratios for the level I elements;
there were 20 such materials. The multiplicity of the
set of features was 41, because we augmented the
previous set with the time_AR feature. Figure 6
shows the chart of ARs for that population.
The charts in Figure 4, Figure 5, and Figure 6
prove the thesis that the structure of an e-learning
material has a strong effect on its quality. Therefore,
we conclude that the existence of specific elements,
the assessment of the quality of each such element,
and the time ratio for the level I elements can be
used as partial measures for the quality.
The next phase is to select a sufficient subset of
features that can be used to estimate the quality of e-
learning materials.
MEASURES FOR ESTIMATING THE QUALITY OF E-LEARNING MATERIALS IN THE DIDACTIC ASPECT
207