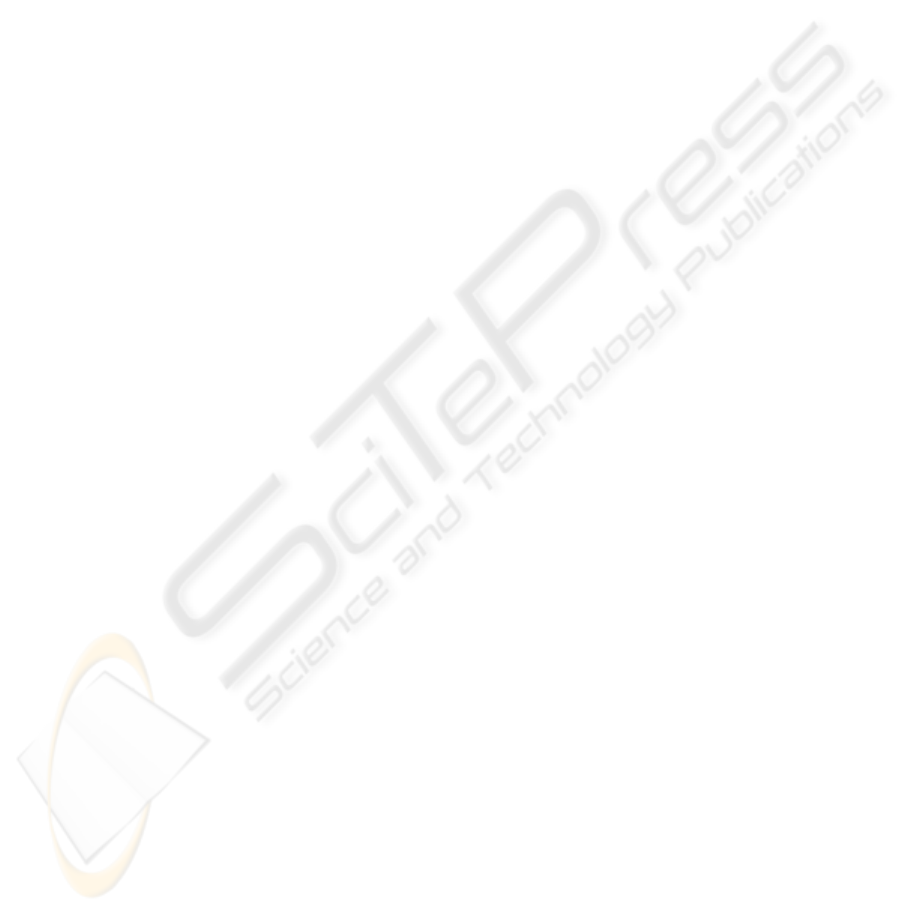
the one hand we look at the perspective: internal
(management, employees) and external (customer,
society); on the other hand we classify indicators as
short-term or long-term. We identify the cost of
measurement of an indicator as essential in choosing
whether an indicator is eligible for pre-ante
monitoring and analysis. We have validated our
framework with a domain expert, and have planned
multiple case-studies and interviews for validation
as future work.
Other directions for future work include
obtaining more insight in the relationships between
the indicators as well as the relationships between
indicators on different aggregation levels. The
knowledge gained will be applied in the DEAL
project – which aims at the development of an
agent-based software system for road-distribution
planning. In such a system we represent the involved
logistical parties as agents operating within a multi-
agent system. In order to give the agents the proper
decision objectives, insight in logistical KPIs is
needed. Finally, we are currently developing a
formal language for expressing the relationships
between the indicators and reasoning about these,
drawing inspiration from the field of requirements
engineering.
ACKNOWLEDGEMENTS
This work is part of DEAL (Distributed Engine for
Advanced Logistics) supported as project
EETK01141 under the Dutch EET programme. For
this particular paper we are very grateful for the
contributions of Jos van Hillegersberg, Peet van
Tooren, Jan Treur, Steef van de Velde, and Pinar
Yolum.
REFERENCES
Brewer, P. C. & Speh, T. W. (2000). Using the balanced
scorecard to measure supply chain performance.
Journal of Business Logistics 21(1):75-93.
Bromley, P. (2001). A Measure of Logistics Success.
Logistics Quarterly 7(3).
Chapman, R. L., C. Soosay, Kandampully, M. (2003).
"Innovation in logistics services and the new business
model." International Journal of Physical Distribution
and Logistics Management 33(7): 630-650.
Christopher, M (1998) Logistics and Supply Chain
Management: strategies for reducing cost and
improving service 2nd Edition. Financial Times /
Prentice-Hall, London.
Christopher, M., & Towill, D. R. (2002). Developing
Market Specific Supply Chain Strategies. International
Journal of Logistics Management, 13(1): 1-14.
Donselaar, K. v., Kokke, K. and Allessie, M. (1998).
Performance measurement in the transportation and
distribution sector. International Journal of Physical
Distribution & Logistics Management 28(6): 434-450.
Fisher, M. L. (1997). What is the Right Supply Chain for
your Product? Harvard Business Review
(March/April): 105-116.
Fowkes, A. S., P. E. Firmin, et al. (2004). How Highly
Does the Freight Transport Industry Value Journey
Time Reliability - and for What Reasons?
International Journal of Logistics - Research and
Applications 7(1): 33-43.
Gibson, B. J., S. M. Rutner, et al. (2002). Shipper-carrier
partnership issues, rankings and satisfaction.
International Journal of Physical Distribution and
Logistics Management 32(8): 669-681.
Graham, T. S., dougherty, P. J., & Dudley, W. N. (1994)
The long term strategic impact of purchasing
partnerships. International Journal of Purchasing and
Materials Management, 32(4): 797-805.
Gunasekaran, A., Patel, C. and Tirtiroglu, E. (2001).
Performance measures and metrics in a supply chain
environment. International Journal of Operations &
Production Management 21(1/2): 71-87.
Hammer, M. (2001). The superefficient company.
Harvard Business Review 79(8): 82.
Ittner, C. D., & Larcker, D. F. (2003) Coming Up Short on
Nonfinancial Performance Measurement. Harvard
Business Review, 81(11): 88-96.
Kaplan, R. S., & Norton, D. P. (1992). The Balanced
Scorecard - Measures that Drive Performance.
Harvard Business Review 75(2): 70-79.
Kemppainen, K. and A. P. J. Vepsaelaeinen (2003).
Trends in industrial supply chains and networks.
International Journal of Physical Distribution and
Logistics Management 33(8): 701-719.
Knemeyer, A. M., Corsi, T. M., & Murphy, P. R. (2003).
Logistics outsourcing relationships: Customer
perspectives. Journal of Business Logistics, 24(1):77-
110.
Lai, K.H., Ngai, E.W.T., Cheng, T.C.E. (2004), An
empirical study of supply chain performance in
transport logistics, International Journal of
Production Economics 87: 321-331.
Lemoine, W. and L. Dagnaes (2003). Globalisation
strategies and business organisation of a network of
logistics service providers. International Journal of
Physical Distribution and Logistics Management
33(3): 209-228.
Lewis, I., Talalayevsky, A. (2000). Third-Party Logistics:
Leveraging Information Technology. Journal of
Business Logistics; 21, 2: 173-185
Lohman, C., Fortuin, L., Wouters, M. (2004). Designing a
performance measurement system: A case study.
ICEIS 2005 - ARTIFICIAL INTELLIGENCE AND DECISION SUPPORT SYSTEMS
246